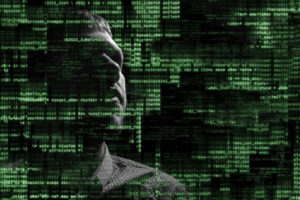
I’ve written before that security is fundamentally an information management problem. It’s about having good sensors and instrumentation in the environment, having that information flow to a central repository where anomalies can be identified, and then being able to take action on it back in the environment. That’s traditionally be done through a SIEM solution, and while they have provided significant advances to our security posture, we need to look ahead to more sophisticated defenses – to move beyond signatures and rules, to behavior.
Endpoint antimalware has undergone a similar transition. We all started out running signature based antivirus, which was pretty effective – in the early 2000’s – at protecting us against known threats. Within a short time, most of the large vendors have about the same hit rate, so it’s became an arms race to see which vendor can update their signatures fastest as the competitive differentiator. That’s why many programs I work with are moving towards the ‘free’ solutions bundled in with the operating system, particularly on the latest OS releases, and then redeploying that spend elsewhere.
Elsewhere is to modern antimalware solutions focused on the behavior of the system. Attacks have patterns of behavior, so they have rules that aren’t based on a simple file hash, but are still common across all users. Having a web browser open a new window minimized, then have constant traffic even while minimized, is a pretty good indication that something is up, even if the destination IP address hasn’t shown up as a bad on a threat feed.
Security analytics systems have followed a similar path. Initially they focused on catching signatures – IP’s, domains, URLs, hashes that were known IOCs. Later, they started aggregating information across multiple sources to cross-correlate activity and alert on it. Downloading information from the payroll system, followed by Dropbox activity to a non-approved account, for example. Data activity monitoring or DLP plus CASB, brought together in a SIEM catches those kinds of attacks. Don’t get me wrong, this is a huge advance in capability, and catches a large number of attacks early in the kill chain. Yet it falls short when we’re trying to defend against advanced, unknown attack vectors.
Most modern analytics platforms have started to use AI and machine learning to create individual user profiles. The above example might be appropriate for a given role or individual, but not for others. These user-level capabilities allow us to assign risk scores to accounts, but the models are still fairly limited. Most rely on behavior over the previous several weeks or a few months. They’re also largely focused on human users of systems, and ignore entities like bots, servers, or containers…let alone televisions, toasters, and other IOT devices.
That’s the first analytics advance, and the one closest to mainstream. To move beyond user behavior analytics, towards true entity analytics for everything in the environment. A lot of that can be automated – platforms can build and maintain behavior models based on past (assumed good) behavior, and then detect deviations from that norm. Yet those too have limits, as they’re generic for a class of entity across multiple organizations.
That’s the second advance: inserting business cycle knowledge into behavior models. An online retailer probably has a good idea of what their container workload profile looks like. If they see a CPU or traffic spike, they can infer that something has gone wrong (either a incident, or an IT issue), and take the container down – ideally automatically. But if that happened on Black Friday, it wouldn’t be an anomaly.
That’s an obvious example, but let’s take others. We see a flurry of use of box.net right before the end of a quarter. Alert? If it’s coming from the folks doing our M&A work, maybe. But if it’s a sales rep, communicating new pricing to a customer using the customer’s file sharing solution (rather than ours), probably not. In fact, if our team blocks that, the VP of sales is probably going to have words with our CISO. I could go on, but you see my point. We’re going to need to bake business knowledge into our models. At the minimum, that probably means a 12 month behavior window for most entities, with the business calendar being one of the factors included in the model.
The last advance on the horizon is deploying the ability to build machine learning models directly into the hands of the defenders. For most organizations, the idea of a data scientist who understands security use cases and can build a model is beyond their reach – a very expensive purple squirrel as one recruiter described it. So we need to make it easy for a defender to build and deploy a model that’s customized to the environment – to merge AI/ML with the existing rule capabilities in our analytics platforms, and alert on events specific to our critical assets and their unique behavior across the annual business cycle.
And that’s the evolution of SIEM we’re headed towards. Not just a security platform, but a business security analytics platform. Yes, we’re still going to need signatures and rules, as well as automatic and generic behavior analytics. That’s where most of our threats are. For the true APT’s though, we need far more dynamic, flexible and mass customized business aware AI and ML to improve our chance of detecting them before the boom happens.