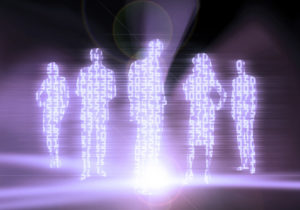
There’s a ton of hype about cognitive security in the marketplace these days, and the marketing departments are operating in full force. So beyond the hand waving, cheerleading and me-too-ing, what do we actually mean by cognitive?
Cognitive involves three things: The ability to mine data for information, the ability to recognize patterns in that data, and the ability to understand natural language. The key component across all of these is an ability to reason and infer on a probabilistic basis from the context of the information. But it’s not Lt. Commander Data from Star Trek fame – cognitive isn’t artificial intelligence. It’s more like the library computer in the original series, that is, a machine that can answer questions put to it. Cognitive is a foundational technology for AI, but we’re a long way from real AI – 2001 came and went without HAL, and so will 2017.
Machine learning, which is often confused with cognitive (sometimes deliberately) has been around for years, and while it’s an enabling technology, there’s no magic there. It can be extremely useful, but also some limitations to keep in mind. The models created are only as good as the data inputs and variables selected. Poor input data yields models that may appear to work, but diverge over time, and you’d best hope that the data isn’t already compromised when the model is built. Even when you have a good baseline, continuously updated models can be either spoofed (reset the ‘normal’ baseline over time), or destabilized by a persistent, and patient attacker. There’s techniques to combat the attacks, so it’s worth asking about which ones are used.
Cognitive uses machine learning as a training tool when it’s being taught to understand a particular set of vocabulary and grammar – cybersecurity for example. Traditional unstructured information systems simply operate on keywords and often metadata, but cognitive systems understand the context of the information components in relation to each other. For example, if I talked about Apple’s CEO eating an apple while negotiating a contract with Apple, most engines would return the document based on a keyword – Apple, or potentially from tags or metadata a human added to the document. A cognitive engine with a large corpus might return that document for questions about computer companies, fruit that grows on trees, and the Beatles’ record company, depending on how the question was worded.
So when using terms like machine learning, cognitive, or artificial intelligence applied to cyber security, it’s important to be crisp about which one is used, and what it implies. We’re not quite in snake oil territory here, but there is a lot of both intentional fuzziness and casual laziness in the press and marketing. Regardless of which term though, remember that there’s no silver bullet that will solve your security challenges. Cognitive is a force multiplier, but not a magic army.